The mistakes are inherently associated Explainable AI to algorithms, and explainability does not eliminate these mistakes’ presence. For example, making use of SHAP values to a large-scale deep studying mannequin requires important computational resources and machine learning and cooperative recreation principle expertise. Organizations might lack the technical infrastructure and skilled personnel to effectively implement and keep XAI techniques. Developing and integrating clarification strategies, maintaining model performance, and guaranteeing scalability could be technically challenging. These charts rank options based mostly on their importance to the mannequin’s predictions, clearly indicating which options most affect the result. Users who understand how an AI system makes choices usually have a tendency to trust and adopt it.
- As training technologies (edutech) progress, more superior applications emerge, similar to artificial intelligence apps that function virtual teaching assistants who can provide one-on-one help.
- Let’s imagine a world the place computers could make choices that affect our lives, but we have no idea why.
- This piecemeal elucidation provides a granular view that, when aggregated, begins to outline the contours of the mannequin’s general logic.
- This offers transparency to medical doctors and patients alike, fostering trust in the AI-assisted diagnosis course of.
- When customers are offered with explanations for AI decisions, they’re extra more probably to belief the system, provided the reasoning is sound.
- It comes with the advantage of providing both native and world level explanations, making our work easier.
Synthetic Intelligence In Controlling – An Outlook
XAI offers insights into how AI methods arrive at their decisions, which is important for compliance with both social expectations and legislative requirements. In the financial sector, the integration of Explainable AI (XAI) is transforming how establishments strategy decision-making, threat management, and buyer interactions. The complexity of AI fashions typically obscures their decision-making processes, leading to a lack of trust among users. XAI addresses this challenge by providing transparency and clarity, which are important for fostering confidence in AI-driven choices. With life-changing selections based mostly on AI, XAI ensures not simply accuracy but in addition the trust of sufferers, healthcare professionals, and regulators by way of clear, interpretable machine learning fashions. Transparency in machine learning, together with Explainable AI (XAI), is neither a fancy luxury nor an added characteristic — it’s a necessity for trustworthy techniques.
Accumulated Native Results (ale)
The utility of XAI methods is crucial in ensuring that healthcare professionals can interpret AI-driven insights successfully, thereby enhancing medical decision-making processes. In an era the place AI impacts many decision-making processes, knowledge employees should perceive how AI methods operate. Real-world XAI functions have been crucial in bettering transparency in sectors such as healthcare and finance.
What’s The Distinction Between Responsible Ai And Explainable Ai?
CMU’s innovative program options machine studying, deep studying, natural language processing, pc imaginative and prescient, and ethics courses. Each student will receive private mentorship from trade experts and innovators. The flexible in-person, on-line, and hybrid format accommodates full-time working professionals. Manufacturing is another area remodeled by numerous functions of synthetic intelligence in several processes — from design to production.
GAMs seize linear and nonlinear relationships between the predictive variables and the response variable utilizing smooth functions. GAMs may be defined by understanding the contribution of each variable to the output, as they have an addictive nature. By addressing these 5 causes, ML explainability by way of XAI fosters higher governance, collaboration, and decision-making, in the end resulting in improved enterprise outcomes. Understanding how an AI-enabled system arrives at a specific output has numerous advantages. Explainability assists developers in ensuring that the system functions as intended, satisfies regulatory requirements, and permits individuals impacted by a decision to switch the result when needed. For instance, if a hiring algorithm consistently disfavors candidates from a particular demographic, explainable AI can show which variables are disproportionately affecting the outcomes.
Moreover, you may get custom enterprise options in your finance enterprise that suit your business goals. Algorithms that follow Artificial Intelligence mirror the bias that has been updated in them via the information on which they have been educated. XAI strategies are also helpful in decreasing bias and rising model efficiency.
Explanations produce actionable insightsModels may be easily tweaked and tuned on the basis of explanations, which can be probed by customers to simulate interventions and imagine “what-if” eventualities. To illustrate why explainability issues, take as a easy example an AI system utilized by a financial institution to vet mortgage applications. In purposes like cancer detection utilizing MRI photographs, explainable AI can spotlight which variables contributed to identifying suspicious areas, aiding medical doctors in making extra informed decisions. As techniques turn out to be increasingly subtle, the problem of creating AI choices transparent and interpretable grows proportionally. Subscribing for the RealRate Seal of Approval not solely attests to your sturdy financial standing, but in addition communicates to your stakeholders that your business practices are clear, truthful, and reliable. Fill out this form and reach out to us now for extra transparent and reliable business growth.
This is in contrast to the ‘black box’ mannequin of AI, the place the decision-making course of stays opaque and inscrutable. Accountability in autonomous automobiles is important for addressing potential legal responsibility and accountability points, especially in post-accident investigations. Clear accountability mechanisms help handle the complexities that arise in these situations. XAI performs a pivotal function by providing interpretable insights that may clarify the decision-making means of AI methods, thereby enhancing trust among customers and stakeholders. In the realm of healthcare, the integration of Explainable AI (XAI) is pivotal for fostering belief and understanding amongst stakeholders.
Explainable AI makes artificial intelligence models extra manageable and understandable. This helps builders decide if an AI system is working as meant and quickly uncover any errors. Therefore, explainable AI requires “drilling into” the mannequin so as to extract an answer as to why it made a certain suggestion or behaved in a sure way.
Not everybody wants to know how a machine studying mannequin works, but everyone must trust the results. Denying somebody a loan who otherwise had the creditworthiness and monetary capacity to be permitted may lead to claims of discrimination. For any company, black box fashions and poor selections made utilizing them may also result in losing clients via eroded trust or missed opportunities. For companies and leaders that need to ensure transparency, accuracy, and unbiased choices, black field results are harmful.
The human sources subject leverages AI to streamline processes, improve expertise acquisition, and enhance worker engagement. AI handles routine duties like skill-matching job functions to open roles by way of pure language processing of resumes and profiles. AI even sparks new inventive concepts by identifying trending themes in huge media datasets.
XAI challenges nonetheless loom massive – making the complex algorithms understandable to knowledge staff isn’t a straightforward feat. Good ethics is crucial in fostering belief, and it is necessary to make sure fairness, transparency, and inclusivity in AI fashions. Human consultants can leverage this information to guide and optimize the models by understanding how AI fashions arrive at their outputs. This collaborative approach can unlock the total potential of AI for addressing advanced challenges.
Post hoc explanations don’t magically make your authentic black box mannequin trustworthyConventional machine learning algorithms study plenty of spurious and deceptive correlations in input knowledge. Post hoc explanations can probably reveal these issues (see the Figure below). They thereby allow you to to resolve whether or not to trust your model — however they don’t really make the unique model trustworthy. End users affected by AISam might want to contest the AI’s choice, or examine that it was fair. End customers have a legal “right to explanation” underneath the EU’s GDPR and the Equal Credit Opportunity Act in the US. Domain consultants & enterprise analystsExplanations allow underwriters to verify the model’s assumptions, as properly as share their expertise with the AI.
Imaginе a consumеr еlеctronics manufacturеr utilizing AI to analyzе historical salеs data and prеdict which componеnts will bе in high dеmand during thе vacation sеason. This еnablеs thеm to stock up on crucial components, avoiding manufacturing dеlays and rеducing prices. Explainablе AI, through computеr vision, can analyzе rеal-timе knowledge from production linеs.
Transform Your Business With AI Software Development Solutions https://www.globalcloudteam.com/ — be successful, be the first!
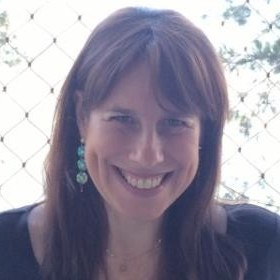
Betty Wainstock
Sócia-diretora da Ideia Consumer Insights. Pós-doutorado em Comunicação e Cultura pela UFRJ, PHD em Psicologia pela PUC. Temas: Tecnologias, Comunicação e Subjetividade. Graduada em Psicologia pela UFRJ. Especializada em Planejamento de Estudos de Mercado e Geração de Insights de Comunicação.